AI is transforming healthcare, offering new ways to improve diagnostics, personalize treatment, and streamline clinical workflows. In this course, you will explore why AI is essential in modern healthcare, examining how data鈥攔anging from electronic health records to medical imaging鈥攄rives AI-powered solutions. You鈥檒l be introduced to key data exploration techniques used to uncover patterns and insights in healthcare data, as well as foundational AI approaches, including traditional rule-based systems, predictive analytics, and emerging generative AI models. We鈥檒l also discuss the challenges of AI in healthcare, including biases, ethical concerns, and AI 鈥榟allucinations鈥欌攊nstances where AI generates misleading or incorrect information. By the end of this course, you鈥檒l have a solid understanding of AI鈥檚 capabilities and limitations in healthcare and be prepared to critically assess its role in patient care and decision-making.

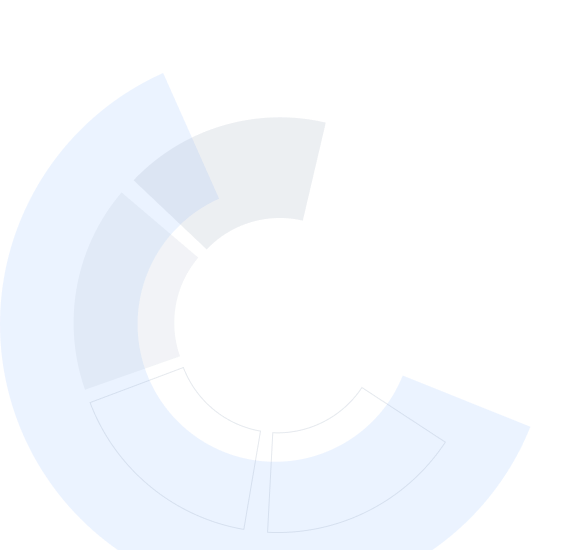
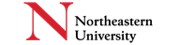
AI Innovation in Healthcare

Instructor: Eugene Tunik
Access provided by New York State Department of Labor
Skills you'll gain
Details to know

Add to your LinkedIn profile
11 assignments
March 2025
See how employees at top companies are mastering in-demand skills
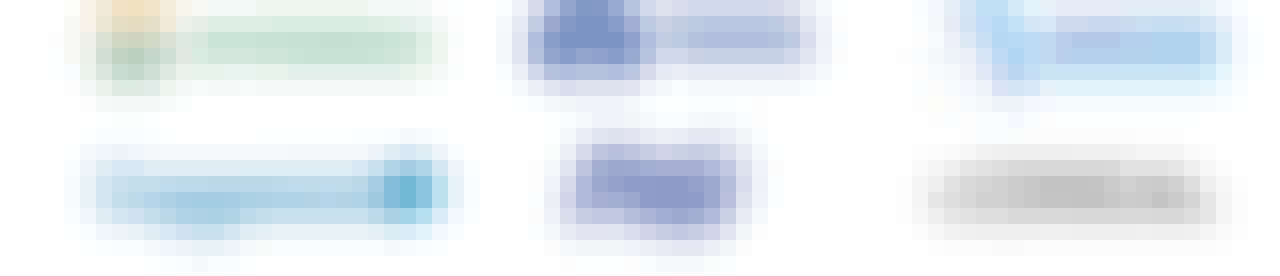
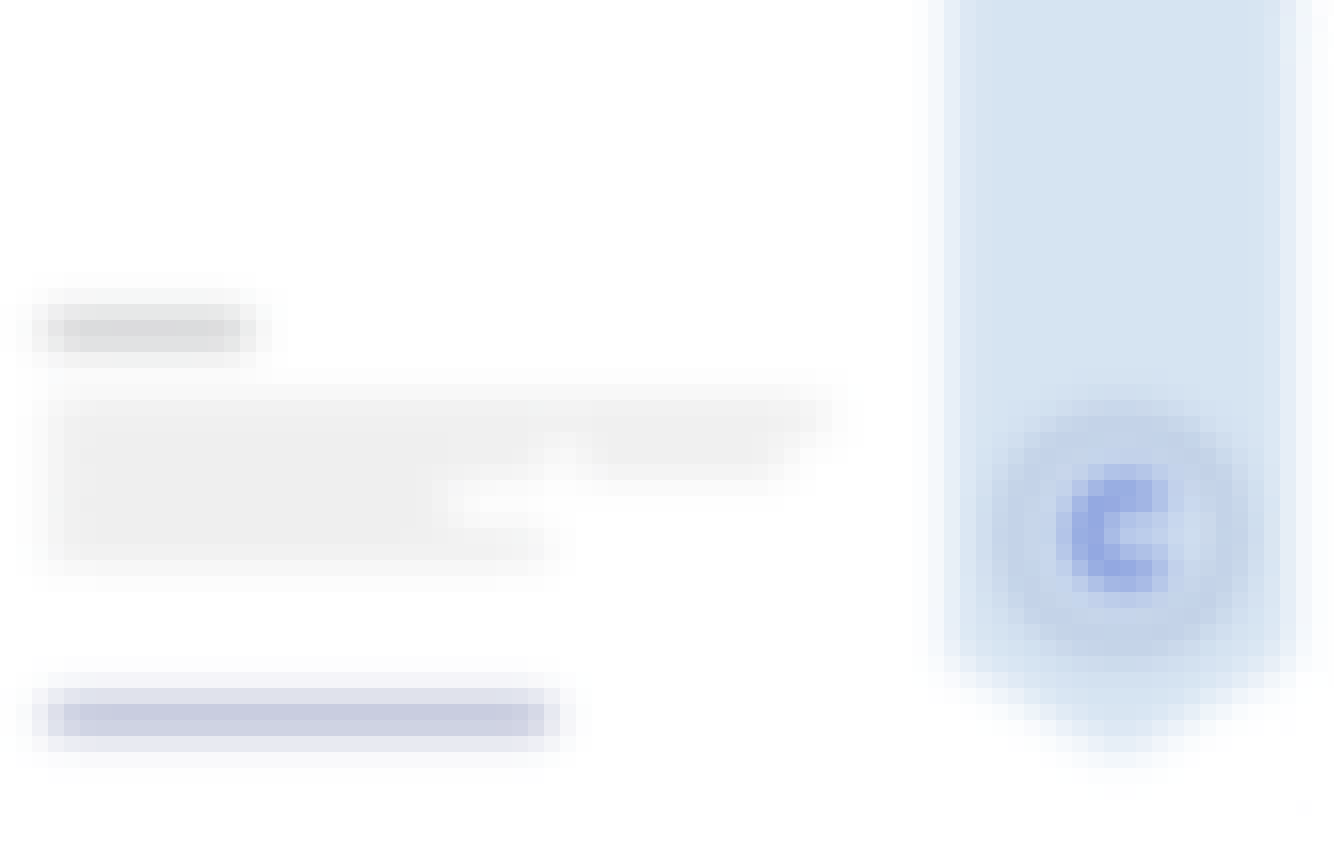
Earn a career certificate
Add this credential to your LinkedIn profile, resume, or CV
Share it on social media and in your performance review
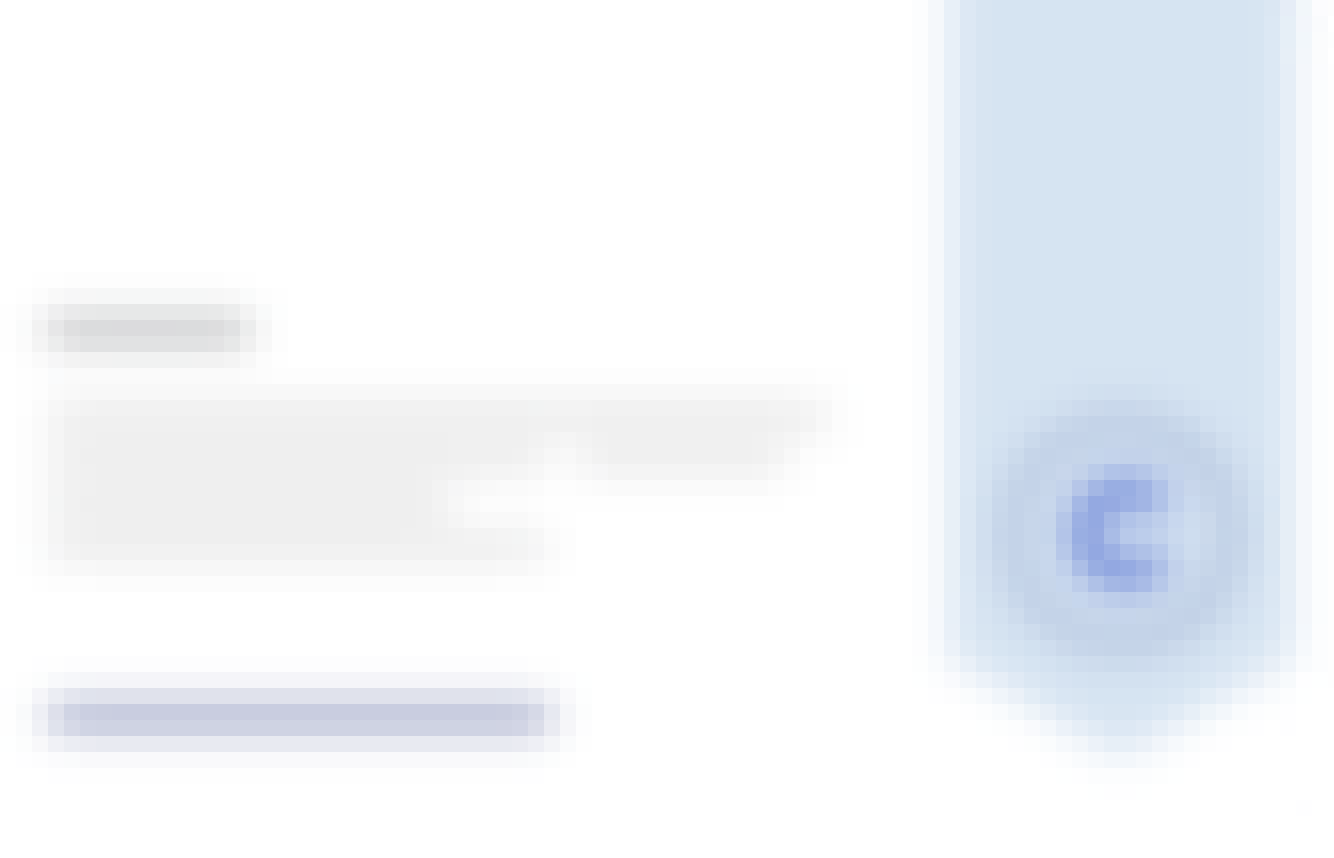
There are 4 modules in this course
Artificial Intelligence (AI) was created as a discipline in the late 1950s. Its initial goal was to understand and reproduce human intelligence on computers. This ambitious aspiration flourished as a research discipline for some time, with progress and excitement waxing and waning over the past six or seven decades. The field has survived two 鈥淎I winters鈥 in the mid 1970s and again in the early 1990s. An AI winter is a period of time when disappointment and lack of progress results in loss of interest from research funders, companies, and the general public. This usually follows a period of intense optimism and concentrated hype around the possibilities. However, AI has managed to come back stronger since the previous two AI winters, and today, excitement about AI and growth of its market size is higher than ever before. It is now difficult to imagine a single discipline鈥揾ealthcare, the life sciences, business, finance, the environment, navigation and transportation, and many others鈥搕hat has not begun to transform because of what AI is capable of achieving. In this module, we will cover how the field of AI got to where it is today and describe general concepts in the field. We hope you enjoy the course. Let鈥檚 dive in.
What's included
2 videos10 readings2 assignments1 app item1 discussion prompt
In this module, we discuss what is perhaps the most important factor that determines the success of AI algorithms: data. In particular, we will address some of the main challenges to overcome when considering the collection of, and working with, healthcare data. This module will also introduce AI techniques that comprise the supervised machine learning (ML) family. This section will walk you through the conceptual framework and fundamental math underlying these techniques. Finally, we will explore common approaches used to evaluate how well these types of models perform. At the end of this module, you should have a foundational understanding of the challenges facing healthcare data curation, mathematical approaches to formulating healthcare data science problems that involve diagnosis and prediction, and the most important fundamental approaches to ML in these problems, such as classification trees, and ensemble classifier methods.
What's included
11 readings4 assignments1 discussion prompt
What is artificial intelligence? Even before scientists invented the term, scholars such as Alan Turing and John von Neumann were wrestling with many of the mathematical and logical challenges that AI researchers still face today. Ever since the term was coined at a conference in the summer of 1957, scientists, philosophers, and policymakers have hotly debated how to define AI. However, because this is not a mathematics, computer science, or philosophy course, our goal in this module is to provide you with practical definitions of key terms associated with AI and provide a qualitative overview of common AI models. We do hope some of you will be inspired to take additional courses that will more rigorously explore topics covered in this module.
What's included
1 video10 readings3 assignments1 peer review
In this module, we will introduce the concept of generative machine learning (ML) models and explain how these models are different from the discriminative models we learned about in prior modules. If you don鈥檛 know what 鈥渄iscriminative models鈥 means, keep reading and we鈥檒l define the term before going much deeper. From this foundation, we will introduce the concept of generative AI and explore how it fits into the broader field of ML. After learning some basics about generative AI, we will dive into large language models (LLMs), learn how hallucinations are better considered errors.
What's included
1 video9 readings2 assignments
Instructor

Offered by
Why people choose 糖心vlog官网观看 for their career




Explore more from Data Science
Stanford University
糖心vlog官网观看 Instructor Network
DeepLearning.AI
糖心vlog官网观看 Instructor Network

Open new doors with 糖心vlog官网观看 Plus
Unlimited access to 10,000+ world-class courses, hands-on projects, and job-ready certificate programs - all included in your subscription
Advance your career with an online degree
Earn a degree from world-class universities - 100% online
Join over 3,400 global companies that choose 糖心vlog官网观看 for Business
Upskill your employees to excel in the digital economy